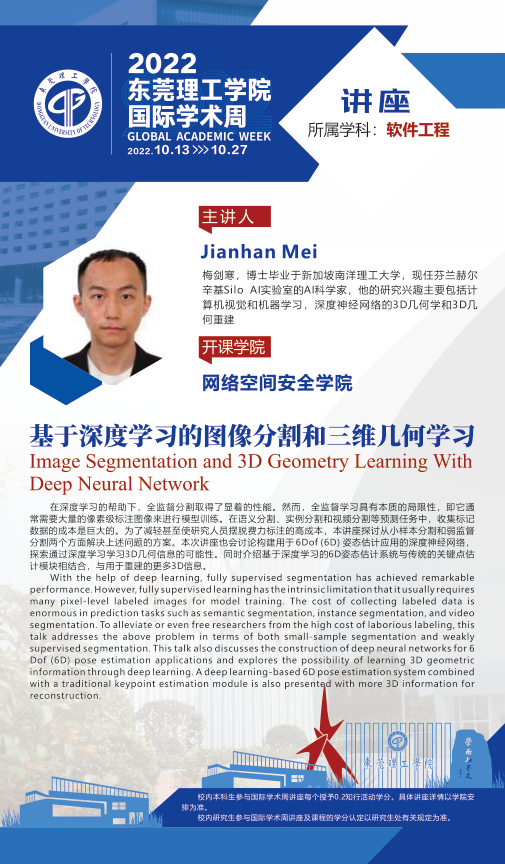
GAWS202223 Image Segmentation and 3D Geometry Learning with Deep Neural Network 基于深度学习的图像分割和三维几何学习
国际学术周讲座ID:GAWS202223
开课学院:网络空间安全学院
讲座名称:Image Segmentation and 3D Geometry Learning with Deep Neural Network 基于深度学习的图像分割和三维几何学习
上课时间安排表:以院系具体通知开课时间为准
课程学位数:100
授课平台:腾讯会议
讲座简介:
With the help of deep learning, fully supervised segmentation has achieved remarkable performance. However, fully supervised learning has the intrinsic limitation that it usually requires many pixel-level labeled images for model training. The cost of collecting labeled data is enormous in prediction tasks such as semantic segmentation, instance segmentation, and video segmentation. To alleviate or even free researchers from the high cost of laborious labeling, this talk addresses the above problem in terms of both small-sample segmentation and weakly supervised segmentation. This talk also discusses the construction of deep neural networks for 6 Dof (6D) pose estimation applications and explores the possibility of learning 3D geometric information through deep learning. A deep learning-based 6D pose estimation system combined with a traditional keypoint estimation module is also presented with more 3D information for reconstruction.
在深度学习的帮助下,全监督分割取得了显着的性能。然而,全监督学习具有本质的局限性,即它通常需要大量的像素级标注图像来进行模型训练。在语义分割、实例分割和视频分割等预测任务中,收集标记数据的成本是巨大的。为了减轻甚至使研究人员摆脱费力标注的高成本,本讲座探讨从小样本分割和弱监督分割两个方面解决上述问题的方案。本次讲座也会讨论构建用于 6 Dof (6D) 姿态估计应用的深度神经网络,探索通过深度学习学习 3D 几何信息的可能性。同时介绍基于深度学习的 6D 姿态估计系统与传统的关键点估计模块相结合,与用于重建的更多 3D 信息。
授课教师及简介:
Jianhan Mei, AI Scientist at Silo AI Oy, Helsinki, Finland.
(撰稿:韦艺翔,一审:许鼎,二审:王谦,终审:王际辉)