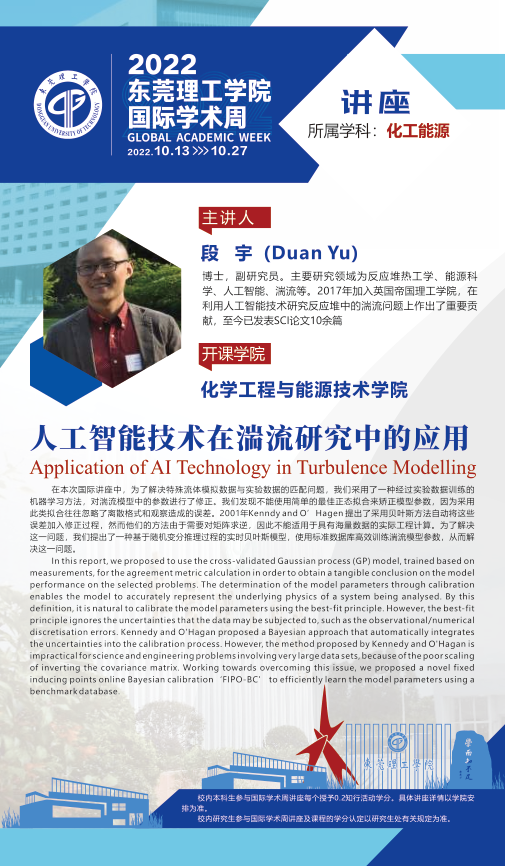
GAWS202216 人工智能技术在湍流研究中的应用 Application of AI technology in turbulence modelling
国际学术周讲座ID:GAWS202216
开课学院:化学工程与能源技术学院
讲座名称:人工智能技术在湍流研究中的应用 Application of AI technology in turbulence modelling
上课时间安排表:以院系具体通知开课时间为准
课程学位数:100-200
授课平台:腾讯视频
讲座简介:
在本次国际讲座中,为了解决特殊流体模拟数据与实验数据的匹配问题,我们采用了一种经过实验数据训练的机器学习方法,对湍流模型中的参数进行了修正。我们发现不能使用简单的最佳正态拟合来矫正模型参数,因为采用此类拟合往往忽略了离散格式和观察造成的误差。2001年Kenndy and O’Hagen 提出了采用贝叶斯方法自动将这些误差加入修正过程,然而他们的方法由于需要对矩阵求逆,因此不能适用于具有海量数据的实际工程计算。为了解决这一问题,我们提出了一种基于随机变分推理过程的实时贝叶斯模型,使用标准数据库高效训练湍流模型参数,从而解决这一问题。
In this report, we proposed to use the cross-validated Gaussian process (GP) model, trained based on measurements, for the agreement metric calculation in order to obtain a tangible conclusion on the model performance on the selected problems [1]. The determination of the model parameters through calibration enables the model to accurately represent the underlying physics of a system being analysed. By this definition, it is natural to calibrate the model parameters using the best-fit principle. However, the best-fit principle ignores the uncertainties that the data may be subjected to, such as the observational/numerical discretisation errors. Kennedy and O'Hagan proposed a Bayesian approach that automatically integrates the uncertainties into the calibration process [2]. However, the method proposed by Kennedy and O'Hagan is impractical for science and engineering problems involving very large data sets, because of the poor scaling of inverting the covariance matrix. Working towards overcoming this issue, we proposed a novel fixed inducing points online Bayesian calibration ‘FIPO-BC’ to efficiently learn the model parameters using a benchmark database [3].
授课教师及简介:
Duan Yu 英国帝国理工学院副研究员
(撰稿:韦艺翔,一审:许鼎,二审:王谦,终审:王际辉)